AI Life2vec
Predicts Mortality
Life2vec is an artificial intelligence model that can predict events in people’s lives with a high degree of accuracy. It is trained using the life details of more than one million people. The model encodes the data in a large system of vectors, a mathematical structure that organizes the different data. It decides where to place data on the time of birth, schooling, education, salary, housing, and health.
In simpler terms, Life2vec is a chatbot that can predict when you will die with 78% accuracy. It can also determine how much money you’ll have when death catches up with you. However, please note that this is a fun approximation and not a scientifically accurate measurement.
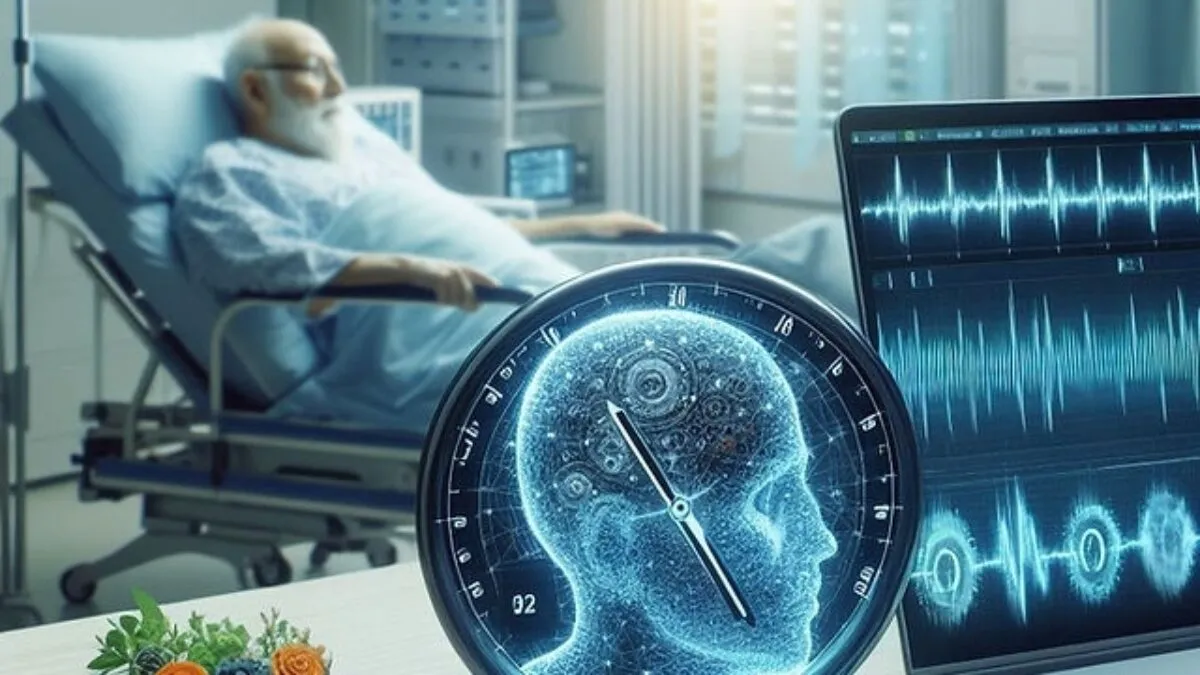
Over the past decade, machine learning has revolutionized computers’ ability to analyze text through flexible computational models.
Due to their structural similarity to written language, transformer-based architectures have also shown promise as tools to make sense of a range of multi-variate sequences from protein-structures, music, electronic health records to weather-forecasts.
We can also represent human lives in a way that shares this structural similarity to language.
From one perspective, lives are simply sequences of events: People are born, visit the pediatrician, start school, move to a new location, get married, and so on.
Here, we exploit this similarity to adapt innovations from natural language processing to examine the evolution and predictability of human lives based on detailed event sequences.
We do this by drawing on arguably the most comprehensive registry data in existence, available for an entire nation of more than six million individuals across decades.
Our data include information about life-events related to health, education, occupation, income, address, and working hours, recorded with day-to-day resolution.
We create embeddings of life-events in a single vector space showing that this embedding space is robust and highly structured.
Our models allow us to predict diverse outcomes ranging from early mortality to personality nuances, outperforming state-of-the-art models by a wide margin.
Using methods for interpreting deep learning models, we probe the algorithm to understand the factors that enable our predictions.
Our framework allows researchers to identify new potential mechanisms that impact life outcomes and associated possibilities for personalized interventions.